Review - (2022) Volume 10, Issue 8
Deep Learning-Based OCT for Epilepsy: A Review
Rukayya Muhammad1, Moussa Mahamat Boukar1, Steve Adeshina2 and Senol Dane3*
*Correspondence: Senol Dane, Department of Physiology, College of Health Sciences, Nile University of Nigeria, Abuja, Nigeria, Email:
Abstract
Automating screening and diagnosis in the medical field saves time and decreases the likelihood of misdiagnosis whilst saving physicians cost and labor time. Deep learning methods development and their feasibility have enabled machines to interpret complex features in medical data, leading to rapid advances in automation. This research discusses how deep learning has been applied to Optical Coherence Tomography in epileptic patients to improve clinical diagnostic categorization, treatment outcome prediction, and comprehension of cognitive comorbidities. It covers the present deep learning research's strengths and weaknesses, as well as the necessity for further studies employing constrained datasets to evaluate the repeatability and generalizability of existing findings, as well as studies to test the clinical utility of deep learning methodologies.
Keywords
Optical Coherence Tomography, Epilepsy, Deep learning
Introduction
Epilepsy is a chronic neurological condition characterized by abnormally high central nervous system activity. It affects approximately 50 million individuals globally, with symptoms ranging from repeated seizures and their physical consequences to numerous psychosocial and mental comorbidities [1]. Appropriate seizure control and medicines for various elements of epilepsy are critical for properly treating people with epilepsy. However, epilepsy exhibits some heterogeneity, which can make it difficult to determine the optimal course of action for every patient. In terms of optimizing clinical diagnostics, prediction, and customized treatment, machine learning algorithms have the potential to surpass traditional approaches.
As the retina is connected to the brain, it has been discovered that changes that occur in the brain in relation to small blood vessels and nerves can be seen in the retina, which is more accessible to image. My research has looked at how the retina changes with epilepsy to investigate what some of these changes are and whether they occur in an earlier stage of the disease. If we know how the retina changes in epilepsy, in the future, an early warning could potentially be flagged at a routine optometrist appointment. Most people with epilepsy have normal MRI scans [2]. It is critical that new technologies are developed to better understand epileptogenesis and to facilitate diagnostic confirmation.
Over the past two decades, Optical coherence tomography (OCT) has gained popularity across a number of medical specialties, notably in ophthalmology and neurology. It offers histologic data on brain tissue in vivo and is a noncontact, no radiation, quick, effective, safe, and repeatable diagnostic imaging method. OCT images and a variety of machine learning methods have been developed to identify abnormalities of the central nervous system (CNS).
Neurology and epileptology are two areas of medicine where deep learning (DL) is a developing trend. Accurate, automated, and quick pattern learning, which may be utilized to design and/or refine therapeutically applicable algorithms for clinical treatment and fundamental research, are three benefits of DL above conventional approaches. Furthermore, DL models incorporating neural networks can handle data increase better than typical ML models. Many image recognition models have been constructed using DL approaches. In contrast to natural RGB visuals, OCT comprises 2D grayscale images and 3D volumes. Furthermore, OCT pictures frequently contain quantitative information that may be used to improve the performance of neural networks. The precise intensity of a pixel, the size of abnormalities, and their position in a scan can all be important clues. Thus, when using DL in OCT pictures, altering the network topologies designed for natural images and accounting for discrepancies might considerably enhance outcomes and push towards clinically viable solutions [3]. Modern OCT image processing techniques, including as retinal layers and avascular region segmentation, disease diagnosis and prognosis prediction, and image quality evaluation, have been greatly enhanced by advances in DL. Different types of traditional OCT input, such as 2D en face pictures, 2D B scans, and 3D volumetric scans, may be used to train DL-based models (Figure 1).
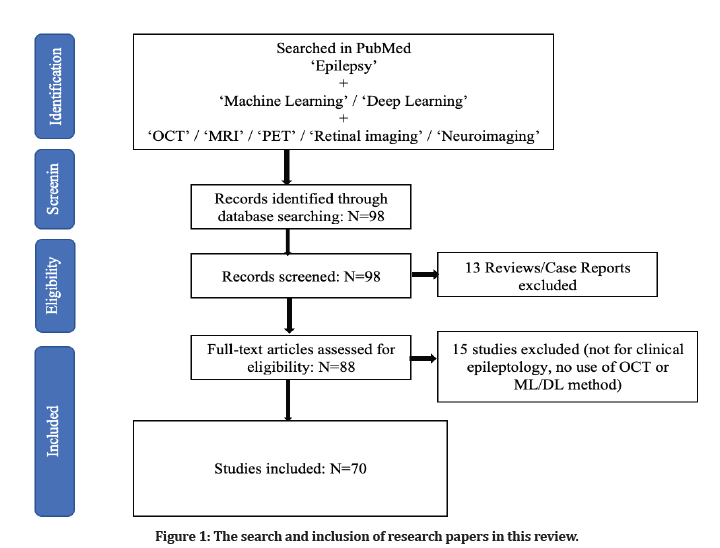
Figure 1: The search and inclusion of research papers in this review.
This paper attempts to give a high-level overview of various modalities by concentrating mostly on the current applications of ML for OCT and other modalities that have been employed in epilepsy. It also highlights potential difficulties with clinical application of DL models and potential future research directions.
Domain knowledge
Epilepsy
Epilepsy is a temporary occurrence of signs and/orf symptoms associated with abnormally excessive or synchronized brain neuronal activity [4]. People may develop epilepsy due to genetic factors, inflammation, infections, changes of normal brain anatomy or factors not yet completely understood [2]. Epilepsy can be broadly divided into focal and generalized, lobe of onset or clinical presentation and underlying neurological disorder [5]. This distinction is helpful as the correct identification of phenotype has implications in diagnosis, investigations, treatment, and, most importantly, prognosis.
Retinal structure
The human eye is an organ that uses light-sensitive tissue to absorb focused light to gather visual information. The retina is the eye's light-sensitive layer. The eye is an organ that uses light-sensitive tissues to absorb focused light to gather visual information. The information sensed by the photoreceptors, which respond to certain spectrum areas of light, is transformed into electrical impulses and sent to the visual cortex of the brain through nerve fibres. The signals are then interpreted as visual images in the brain. There are significant biological markers in the eye. The photoreceptors are heavily concentrated for optimal resolving power in the macula, the centre of the imaging area. The fovea, which is at the macular region's core, is in charge of high-definition central vision. The circular region on the ocular fundus known as the optic disc, also known as the optic nerve head (ONH), is where the nerve fibres that go to the visual cortex converge. The retinal vasculature spreads out from the optic disc to the retinal layers. The retinal layers are classified into seven levels based on the cell kinds [6]. The retinal veins can be classified according to their positions. The retinal veins are categorized based on their location. The nerve fibre layer and the sidewalls of the inner nuclear layers include the superficial, intermediate, and deep retinal arteries. The choroid arteries are found underneath the RPE and Bruch's membrane. The photoreceptors are supplied with nutrients and oxygen via these veins [7].
Optical coherence tomography (OCT)
The optical coherence tomography technique, which uses interferometry to obtain high-resolution cross-sectional pictures of the retina, allows for in-vivo viewing of tissue microstructure. OCT, as a medical imaging modality, is most comparable to ultrasound in that it uses light waves instead of sonic waves. OCT allows for the identification of the retina's cellular layers and the measurement of the thickness of these layers or the retina as a whole, which aids in the early detection and diagnosis of retinal illnesses and disorders. OCT technology has advanced in recent years, allowing it to be applied to a greater number of medical specializations [8]. OCT has been used to perform real-time imaging at a rate of many frames per second. Recent OCT imaging studies have examined developmental biology samples at the cellular level. The interior body may be imaged with OCT using catheters, endoscopes, and laparoscopes. OCT's capacity to give cross-sectional retinal imaging and see the tiny structures in the eye is one of the technology's many medical benefits [9].
Although the retina contains very little optical backscattering, OCT imaging may be quite sensitive, making it possible to observe details with very little backscattering, such as the vitreo-retinal interface. RPE and choroid, on the other hand, stand out in OCT images due to their bright (hyper reflective) appearance. When seen with OCT, the layer of retinal nerve fibres appears as a hyper reflective structure that is thickest at the optic disc and goes thinner as it approaches the fovea. OCT has been utilized to examine retinal laser injuries in addition to studying the retina's dynamic reactions. Intelligent algorithms may be used to quantitatively analyse OCT images, such as determining the thickness of the retinal nerve tissue or the thickness of the retinal nerve fibre layer. The use of OCT, which can offer quantitative data regarding the prognostic assessment used for the diagnosis and monitoring of retinal damage caused by conditions like glaucoma or diabetic macular edoema, is on the rise. Due to its ability to identify and diagnose diseases in their earliest stages before any outward signs or symptoms appear, OCT has been used to prevent irreparable loss of vision [10].
Machine learning methods
The term "machine learning" describes a class of algorithms that may be used in systems designed to tackle issues in a variety of industries. Depending on how the target information must be learnt, it may be divided into two primary categories [11]. The learning process is referred to as supervised learning when there is explicit information or direct human involvement. In the absence of human assistance, learning is referred to as unsupervised or semi-supervised learning, depending on how autonomously the corresponding system interprets the target-related pattern. Unsupervised learning includes k-means clustering, fuzzy c-means (FCM), the Gaussian mixture model (GMM), and reinforcement learning. Supervised learning includes all deep learning techniques that use labels for training, such as k nearest neighbor (kNN), a support vector machine (SVM), fuzzy techniques, and random forest (RF). The existence of parameters in the developed models, or the lack thereof, is another criterion for classifying the kind of learning. The model may be built up with a fixed number of parameters if the data are taken to have a particular distribution. The model has the benefit of being a reasonably simple model because of past information. However, there is less room for improvement when adding new data that does not fit the existing distribution. Bayesian networks, artificial neural networks, logistic regressions, and linear regressions are examples of this type of model. However, the flexibility and somewhat slow processing speed of a non-parametric model created by utilizing the characteristics of data distribution are advantages. These models include the k-nearest neighbor (KNN) classifier, decision trees, and RF [12]. Building neural networks for deep learning is now seen to be an effective way to turn the input data into a condensed representation with end-to-end learning. Depending on the fundamental elements of the architecture and how the nodes are connected, there are several formats for building neural networks. Nodes in the same layer are often not linked to one another since layers are collections of a particular number of nodes. Regarding the fundamental building blocks of a neural network, a fully connected network are one in which every node in one layer is linked to every node in the next layer (FCN). A network is referred to be a convolutional neural network (CNN) if a layer propagates the input by partly connecting to the nodes of the following layer at regular intervals and with the same weights [13]. Furthermore, the network is known as a recurrent neural network (RNN) and is often built to handle sequential data if the inference processes progress by repeatedly feeding the previous outcomes as inputs. Given that deep learning is an advanced technique that is known to outperform traditional machine learning, deep learning models are the main emphasis of the approaches covered in this study. However, if traditional methods can assess the required patterns and produce results that are as excellent as deep learning approaches, they are also covered for some jobs (Figure 2 and Table 1) [14].
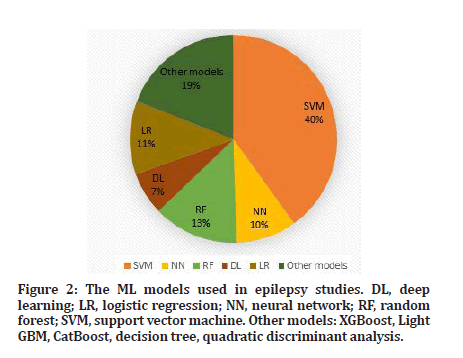
Figure 2: The ML models used in epilepsy studies. DL, deep learning; LR, logistic regression; NN, neural network; RF, random forest; SVM, support vector machine. Other models: XGBoost, Light GBM, CatBoost, decision tree, quadratic discriminant analysis.
References | Subjects | Imaging modality | classifiers | Main outcome |
---|---|---|---|---|
Akeret et al.[14] | 923 brain tumors | T1, FLAIR | DT, GLM, RF, GBM, NN, SVM, GAM | AUC = 0.79, ACC = 0.72 to predict seizure occurrence when combined with clinical info |
Lee et al. [15] | 89 FE (P) | DTI | CNN | ACC = 0.92 to predict functional deficit after surgery |
Wang et al.[16] | 205 LGG-related EPI | T2WI | Novel radiomic nomogram | AUC = 0.863 to predict epilepsy type |
Sinha et al.[17] | 51 TLE, 29 HC | T1, DTI | SVM | AUC = 0.84 to predict seizure outcome after surgery |
Kini et al.[18] | 89 TLE | FDG-PET | RF | ACC = 0.71 to predict seizure outcome after surgery |
ACC: Accuracy; AUC: Area under the ROC curve; DT: Decision tree; DTI: Diffusion tensor imaging; EPI: Epilepsy; FE: Focal epilepsy; GBM: Gradient boosting algorithm; GLM: Generalized linear model; HC: Healthy controls; NN: Neural network; RF: Random forest; SVM: Support vector machine; TLE: Temporal Lobe Epilepsy. |
Table 1: Machine learning applications used to predict clinical outcomes in epilepsy.
There are also various ML applications for more direct associations with clinical outcomes than lesion/focus detection. A major trend is the prediction of postsurgical seizure [13,19–23], in light of the clinical importance. Most of the studies reported 70–90% accuracy for the prediction of seizure outcomes after resection surgery. Other studies presented approximately 85% accuracy for the identification of responders to vagus nerve stimulation (VNS) [24,25]. In terms of surgery, ML methods were also applied and generated good predictive values for postsurgical functional deficit [26], lateralization of the language hemisphere and optimal planning for laser surgery [27] Cognitive dysfunctions in epilepsy were also shown to be predicted by ML methods [5,28,29]. Functional neuroimaging and/or network measurement are often used for this prediction. Other ML applications included predicting drug responsiveness [30], acquiring epileptogenicity [31], and the differentiation of types of autoantibodies.
Deep learning techniques
Deep neural networks are structures with more hidden layers than conventional neural networks, or so-called shallow networks. The number of network parameters increases significantly as networks get bigger, necessitating adequate learning strategies as well as precautions against over fitting the taught network. Convolutional networks drastically reduce the number of trainable parameters by using filters convolved with input patterns rather than multiplying a weight vector (matrix).
Other approaches are also proposed [32] to enable the network in learning. The input pattern size for the subsequent convolutional layer is decreased by pooling layers. Techniques such as batch normalization, dropout, early halting, unsupervised or semi-unsupervised learning, and regularization work to boost learning capacity and speed while preventing the learnt network from over fitting. To prevent over fitting for small amounts of labelled data, the AE and DBN are first used for unsupervised learning and then fine-tuned. RNNs that are able to disclose the long-term time dependencies of data samples include long short-term memory (LSTM) and gated recurrent units (GRU).
Deep learning applications for epilepsy
While deep learning algorithms and tools may not be able to replace visual examination of MRIs by imaging professionals in all circumstances, they may offer crucial support tools and may become very important when such knowledge is not accessible. Some deep learning technologies have already been incorporated into clinical treatment for epilepsy, but for newer ML and DL approaches to be adapted for clinical usage, it will still be crucial for physicians and AI scientists to maintain close communication.
Numerous machine learning techniques have been put forth to automatically classify and identify abnormalities in OCTs [33,34]. These methods often focus on the segmentation of the retinal layer or the categorization of images with similar properties [35]. These techniques provide low-cost, high-performance analytical assistance.
Deep learning–based image quality assessment of OCT
For a more thorough and accurate disease evaluation using OCT images, image quality management is crucial. A DL-based image quality assessment model was created by Ran et al [36] to distinguish between gradable and upgradable OCT optic disc volumetric scans. It performed well in both the two external testing datasets and the internal validation datasets, and it could be used in epilepsy detection models for OCT analysis based on DL. To analyse the quality of OCT scans, Kauer, et al. [37] suggested an automated quality evaluation network based on CNN. The model successfully classified images as good, poor, higher, or lower quality with an accuracy of 99.5%. A multilayer deep CNN was created by Lauermann, et al. [38] for automatic evaluation of the quality of OCT images of the superficial capillary plexus (sufficient vs. insufficient). The DL model's sensitivity, specificity, and accuracy were all over 90.0 percent. Other research used DL to rebuild or denoise OCTA pictures, which significantly improved the image quality and may increase the number of adequate photos, shorten the time it takes to analyse the images, and improve the ability to identify retinal diseases.
The identification of a particular artefact is useful in addition to generic image quality assessment. To identify the capillary NPA from signal reduction artefacts in 6x6mm2 OCTA, Guo, et al. [39] created a CNN-based DL model. A vital quantitative biomarker used in the OCTA assessment of DR is the capillary NPA. For NPA identification, the suggested DL model performed well across a wide range of DR severity and scan quality. It may be able to solve the issue of signal reduction artefacts while measuring capillary dropout in the retina using OCTA.
The differentiation of individuals with epilepsy from healthy controls
The distinguishing of epileptic retinas from healthy retinas is a typical use of machine learning for retina imaging in epilepsy. As shown in Table 2, multiple ML classifiers have achieved above 70% accuracy in distinguishing between persons with epilepsy and healthy controls.
References | Subjects | Imaging modality | classifiers | Main outcome |
---|---|---|---|---|
Del Gaizo et al. [40] | DKI | SVM | ACC = 0.82 for TLE vs. HC by MK | |
Bharath et al. [41] | 42 TLE-HS (18 R, 19 L, 5 B) | rs-fMRI | SVM | ACC = 0.975 for TLE vs. HC. Correlation of network with clinical variables |
Si et al. [42] | 15 JME, 15 HC | HARDI, NODDI | CNN | ACC = 0.752, AUC = 0.839 for JME vs. HC |
Nguyen et al. [43] | 63 DRE (P), 259 HC | rs-fMRI | CNN | ACC = 0.74, AUC = 0.86 for DRE vs. HC |
ACC: Accuracy; AUC: Area under the curve; DKI: Diffusion kurtosis imaging; DT: Decision tree; DTI: Diffusion tensor imaging; EPI: Epilepsy; FE: Focal epilepsy; GBM: Gradient boosting algorithm; GLM: Generalized linear model; HC: Healthy controls; HARDI: High-angular-resolution diffusion imaging; NN: Neural network; RF: Random forest; RS: Resting-state; SVM: Support vector machine; TLE: Temporal lobe epilepsy. |
Table 2: Machine learning applications used to the differentiation of individuals with epilepsy and healthy subjects.
In recent years, ML algorithms using multimodal MRI have been shown to lateralize hippocampal pathology in patients with temporal lobe epilepsy (TLE) and hippocampal sclerosis (HS). A recent ENIGMA-Epilepsy study investigated the performance of ML and DL algorithms using structural MRI and diffusion MRI (dMRI) to classify controls vs patients with TLE with HS andMRI-negative TLE. This study revealed that structural MRI and dMRI-based models had similar accuracy and that models for TLE-HS were more accurate than for MRI negative TLE. While the ability of automatic quantification methods may not currently exceed visual inspection of MRIs by imaging experts in all situations, AI algorithms and tools provide important support tools and may become of great importance when such expertise is not available. Some AI tools have already been integrated into clinical care for epilepsy, but it will remain important for clinicians and AIexperts to remain in close dialog for newer ML and DL approaches to be adapted for clinical use. Focal cortical dysplasia (FCD) is one of the most common causes of pharma coresistant focal epilepsy [14]. However, FCDs are often undetected on conventional MRI and the presurgical diagnosis depends heavily on the expertise of the examiner. Several MRI post-processing techniques have been used to improve the detection of subtle FCDs. A recent Multicentre validated study [4] showed that DL using multimodal MRI data could reliably identify previous MRI-negative FCD lesions, suggesting that DL shows promise for assisting non-expert clinicians in this challenging diagnosis. AI methods can also combine imaging and clinical data to build models for predicting clinical outcomes in patients with epilepsy. For example, automated volumetric MRI measurements incorporated into statistical models help to predict postoperative seizure outcomes in TLE and frontal lobe epilepsy (FLE), revealing that subtle cortical atrophy beyond the surgical resection influences seizure outcome. DL applied to whole brain connectives can also help to predict postoperative seizure control in patients with TLE. Another application of AI has been implemented by the MELD project—a retrospective multicenter cohort of 580 patients with FCD. Here, AI was not used for lesion detection. Rather, the MELD team trained logistic regression models to test for associations between clinical data and the location of FCD lesions that were delineated on T1-weighted MRI scans by imaging experts. Their data-driven atlas validated smaller independent studies which showed a non-uniform distribution of FCDs with higher concentrations in the superior frontal sulcus, frontal pole, temporal pole, and superior temporal gyrus. Lesions in primary sensory areas were associated with earlier age of epilepsy onset whereas lesions in association cortices were associated with a later epilepsy onset. The likelihood of seizure freedom decreased with a longer duration of epilepsy [19]. Finally, AI, including ML and DL, has been applied to neuroimaging data for predicting clinical diagnosis, that is, clinical phenotyping from imaging, and predicting response to antiseizure medications. For example, dMRI measures and connectome profiles may identify patients with juvenile myoclonic epilepsy and distinguish patients with focal epilepsy vs healthy controls. However, these studies are few in number and larger studies that are validated in external datasets are needed to determine.
Discussion And Future Directions
As previously stated, the present ML applications for epilepsy imaging are different in terms of epilepsy disorders addressed, imaging modalities, and ML techniques. Multimodal imaging is a recent trend in epilepsy research, and it has the potential to give complete data [44]. As a result, the number of ML research utilizing different imaging modalities has increased, particularly in recent years. However, each study group appears to have certain preferences for imaging modalities, which may have contributed to the diversity of research in this subject. In terms of ML algorithms, more recent research have tended to employ deep-learning approaches such as CNN [45,46]. The fact that there aren't many research employing unsupervised classification—indeed, just two studies did so [47] is another crucial technique issue. In order to learn more about data-driven knowledge, more research employing unsupervised clustering is required. This approach has the ability to detect hidden patterns in unlabeled data.
Open access OCT data: The absence of OCT data from epileptic patients is a major problem in early-stage prediction and analytic studies. Reproducibility of results depends critically on open access sharing of OCT data from epilepsy databases (using Github or similar repositories). Excessive feature extraction will result in increased computation costs and time requirements.
The categorization of disorders, prognosis monitoring, prediction of functional changes, structural segmentation, and image quality assessment on OCT images were all made possible with the use of DL-based models. ResNet, RNN, and Dense Net, the most widely used networks, were utilised. First of all, the training sample size of the DL models for OCT image processing that were already in use was rather tiny. It may result in model over fitting, a loss in generalizability in unexplored OCT datasets, and a reduction in data diversity. It's helpful to increase the training sample size by using various data augmentation techniques including rotation, flipping, shifting, and cropping. Additionally, recent studies in the field of computer science, such as transfer learning, low-shot learning [48], or few-shot learning [49], also hold promise for resolving this problem. Before using these DL models in real-time clinics, the majority of the research were still in the "proof-of-concept" stage and significant problems needed to be resolved.
The "black box" nature of current DL models, which rely on AI to identify items missed by human sight, limits their interpretability and reliability. To create heatmaps and improve the transparency of DL models, occlusion tests, activation maps, and salient maps were frequently employed. Explainable AI (XAI), which offers tools to expose AI judgments, is increasingly known as a means of opening the "black box." For the OCT segmentation problem in monkey eyes, Maloca et al.81 introduced a CNN that was improved with a post hoc XAI method called Traceable Relevance Explain ability (T-REX). After the neural network training process, post hoc XAI may highlight and show areas of the input data that contribute to pertinent prediction judgments. More work must be done in the future to offer clinics with a real-time illustration of the outcomes of DL-based OCT analysis.
Without sufficient external testing, the majority of DL models for OCT image interpretation were often trained and verified on internal datasets. However, there are fewer open source OCT datasets accessible than there are for retinal fundus images [50], and transferring data between institutions is difficult because of concerns about patient privacy and the need for a lot of storage. The "Model-to-Data" technique, which involves packing a digital learning model that is simple to distribute and bringing it to the data, has been shown to be a viable remedy for the problem of data interchange between various organisations. It could make it possible for a reliable DL model to be built centrally and sent to several clinical locations so that distributed training can take place [51]. Federated learning (FL), a paradigm for learning that allows algorithms to be trained jointly without actually exchanging data, has the potential to solve the issues of data governance and privacy. Each participating university conducts local DL model training, and only attributes (such as parameters and gradients) are shared. Without transferring patient data outside the confines of the institutions where they are housed, it allows for the collaborative acquisition of insights, such as in the form of a consensus model [52].
Although some DL models showed good results in internal validation, there are still important problems for real-world application in various settings (i.e., domain shift) because of the variety of devices and imaging protocols, variations in ocular physiological anatomy, and unbalanced data distribution. The robustness of DL models for medical image analysis is now being proposed to be improved by domain adaption strategies such synergistic fusion and batch normalization [53].
Conclusion
A number of studies have found promising findings using machine learning, which is an emerging trend in the field of retinal imaging in epilepsy. A further difficulty arises from the variety of targeted epileptic syndromes, imaging modalities, feature extractions, and ML algorithms. Deep learning, multimodal imaging, and regression models are current trends, and unsupervised clustering-based studies should be used in future research. Consistent upgrading procedures and model improvement are required for improved clinical applications to ensure the models' performance is comparable to that of human competence.
References
- Beghi E, Giussani G, Nichols E, et al. Global, regional, and national burden of epilepsy, 1990–2016: A systematic analysis for the global burden of disease study 2016. Lancet Neurol 2019; 18:357-375.
- Lapalme-Remis SCGD. Imaging for adults with seizures and epilepsy. Contin Lifelong Learn Neurol 2016; 22:1451–1479.
- Raghu M, Zhang C, Kleinberg J, et al. Transfusion: Understanding transfer learning for medical imaging. Adv Neural Inf Process Syst 2019; 32.
- Fisher RS, Boas WV, Blume W, et al. Epileptic seizures and epilepsy: Definitions proposed by the international league against epilepsy (ILAE) and the international bureau for epilepsy (IBE). Epilepsia 2005; 46:470.
- Van Rijckevorsel K. Cognitive problems related to epilepsy syndromes, especially malignant epilepsies. Seizure 2006; 15:227–234.
- Mahabadi N, Al Khalili Y. Neuroanatomy, Retina. StatPearls 2022.
- Kumar V, Abbas AK, Fausto N, et al. Robbins and Cotran pathologic basis of disease, professional edition e-book. Elsevier Health Sciences 2014.
- Podoleanu AG. Optical coherence tomography. J Microsc 2012; 247:209-219.
- Podoleanu AG. Optical sources for optical coherence tomography (OCT). In Lasers for Medical Applications 2013; 253–285.
- Fujimoto JG, Pitris C, Boppart SA, et al. Optical coherence tomography: an emerging technology for biomedical imaging and optical biopsy. Neoplasia 2000; 2:9-25.
- http://machinelearningmastery.com/gentle-introductiongradient- boosting-algorithm-machine-learning.
- Bishop CM. Pattern recognition and machine learning. Springer 2006.
- Sone D, Beheshti I. Clinical application of machine learning models for brain imaging in epilepsy: A review. Front Neurosci 2021; 15.
- Akeret K, Stumpo V, Staartjes VE, et al. Topographic brain tumor anatomy drives seizure risk and enables machine learning based prediction. Neuro Image Clin 2020; 28:102506.
- Lee MH, O’Hara N, Sonoda M, et al. Novel deep learning network analysis of electrical stimulation mapping-driven diffusion MRI tractography to improve preoperative evaluation of pediatric epilepsy. IEEE Trans Biomed Eng 2020; 67:3151-3162.
- Wang Y, Wei W, Liu Z, et al J. Predicting the type of tumor-related epilepsy in patients with low-grade gliomas: A radiomics study. Frontiers Oncol 2020; 10.
- Sinha N, Wang Y, da Silva NM, et al. Structural brain network abnormalities and the probability of seizure recurrence after epilepsy surgery. Neurology 2021; 96:e758.
- Kini LG, Thaker AA, Hadar PN, et al. Quantitative FDG PET asymmetry features predict long-term seizure recurrence in refractory epilepsy. Epilepsy Behavior 2021; 116:107714.
- Sone D, Beheshti I. Clinical application of machine learning models for brain imaging in epilepsy: A review. Frontiers Neurosci 2021; 15.
- Tjepkema-Cloostermans MC, de Carvalho RC, van Putten MJ. Deep learning for detection of focal epileptiform discharges from scalp EEG recordings. Clin Neurophysiol 2018; 129:2191-2196.
- Mutlu U, Bonnemaijer PWM, Ikram MA, et al. Retinal neurodegeneration and brain MRI markers: The rotterdam study. Neurobiol Aging 2017; 60:183–191.
- Tomasi D, Volkow ND. Laterality patterns of brain functional connectivity: Gender effects. Cerebral Cortex 2012; 22:1455-1462.
- Serbecic N, Aboul-Enein F, Beutelspacher SC, et al. High resolution spectral domain optical coherence tomography (SD-OCT) in multiple sclerosis: the first follow up study over two years. PloS One 2011; 6:e19843.
- Hilton EJ, Hosking SL, Betts T. Epilepsy patients treated with antiepileptic drug therapy exhibit compromised ocular perfusion characteristics. Epilepsia 2002; 43:1346-1350.
- Pasol J. Neuro-ophthalmic disease and optical coherence tomography: Glaucoma look-alikes. Curr Opinion Ophthalmol 2011; 22:124–132.
- Gudigar A, Raghavendra U, Samanth J, et al. Automated detection of chronic kidney disease using image fusion and graph embedding techniques with ultrasound images. Biomed Signal Processing Control 2021; 68:102733.
- Wang Y, Wei W, Liu Z, et al. Predicting the type of tumor-related epilepsy in patients with low-grade gliomas: A radiomics study. Frontiers Oncol 2020; 10.
- Lado FA LE and MS. Seizure-induced hippocampal damage in the mature and immature brain. Epileptic Disord 2002; 4:83–97.
- Sharma M, Rajput JS, Tan RS, et al. Automated detection of hypertension using physiological signals: A review. Int J Environ Res Public Health 2021; 18:5838.
- Mo J, Liu Z, Sun K, et al. Automated detection of hippocampal sclerosis using clinically empirical and radiomics features. Epilepsia 2019; 60:2519–2529.
- Paldino MJ, Hedges K, Zhang W. Independent contribution of individual white matter pathways to language function in pediatric epilepsy patients. NeuroImage Clin 2014; 6:327–332.
- Goodfellow I. Deep learning. Cambridge, UK, MIT Press 2016.
- Wang J. OCT image recognition of cardiovascular vulnerable plaque based on CNN. IEEE Access 2020; 8:140767–140776.
- Raja H, Akram MU, Shaukat A, et al. Extraction of retinal layers through convolution neural network (CNN) in an OCT image for glaucoma diagnosis. J Digital Imag 2020; 33:1428–1442.
- Awais M, Müller H, Tang TB, et al. Classification of sd-oct images using a deep learning approach. In 2017 IEEE International Conference on Signal and Image Processing Applications (ICSIPA) 2017; 489-492.
- Ran AR, Shi J, Ngai AK, et al. Artificial intelligence deep learning algorithm for discriminating ungradable optical coherence tomography three-dimensional volumetric optic disc scans. Neurophotonics 2019; 6:041110.
- Kauer J, Gawlik K, Zimmermann HG, et. al. Automatic quality evaluation as assessment standard for optical coherence tomography. In: Mahadevan-Jansen A, Advanced Biomedical and Clinical Diagnostic and Surgical Guidance Systems 2019.
- Lauermann JL, Treder M, Alnawaiseh M, et al. Automated OCT angiography image quality assessment using a deep learning algorithm. Graefe's Arch Clin Exp Ophthalmol 2019; 257:1641-1648.
- Guo Y, Hormel TT, Xiong H, et al. Development and validation of a deep learning algorithm for distinguishing the nonperfusion area from signal reduction artifacts on OCT angiography. Biomed Optics Express 2019; 10:3257-3268.
- Del Gaizo J, Mofrad N, Jensen JH, et al. Using machine learning to classify temporal lobe epilepsy based on diffusion MRI. Brain Behav 2017; 7:e00801.
- Bharath RD, Panda R, Raj J, et al. Machine learning identifies “rsfMRI epilepsy networks” in temporal lobe epilepsy. Eur Radiol 2019; 29:3496–3505.
- Si X, Zhang X, Zhou Y, et al. Automated detection of juvenile myoclonic epilepsy using CNN based transfer learning in diffusion MRI. IEEE Eng Med 2020.
- Nguyen RD, Kennady EH, Smyth MD, et al. Convolutional neural networks for pediatric refractory epilepsy classification using resting-state functional magnetic resonance imaging. World Neurosurg 2021; 149:e1112–e1122.
- Sone D, Beheshti I, Maikusa N, et al. Neuroimaging-based brain-age prediction in diverse forms of epilepsy: a signature of psychosis and beyond. Molecular Psychiatr 2021; 26:825–834.
- Nguyen RD, Kennady EH, Smyth MD, et al. Convolutional neural networks for pediatric refractory epilepsy classification using resting-state functional magnetic resonance imaging. World Neurosurg 2021; 149:e1112–e1122.
- Taylor PN, Sinha N, Wang Y, et al. The impact of epilepsy surgery on the structural connectome and its relation to outcome. Neuro Image Clin 2018; 18:202–214.
- Zhang L, Wang M, Liu M, et al. A survey on deep learning for neuroimaging-based brain disorder analysis. Front Neurosci 2020; 14.
- Burlina P, Paul W, Mathew P, et al. Low-shot deep learning of diabetic retinopathy with potential applications to address artificial intelligence bias in retinal diagnostics and rare ophthalmic diseases. JAMA Ophthalmol 2020; 138:1070-10777.
- Yoo TK, Choi JY, Kim HK. Feasibility study to improve deep learning in OCT diagnosis of rare retinal diseases with few-shot classification. Med Biol Eng Comput 2021; 59:401–415.
- Khan SM, Liu X, Nath S, et al. A global review of publicly available datasets for ophthalmological imaging: Barriers to access, usability, and generalisability. Lancet Digital Health 2021; 3:e51-66.
- Mehta N, Lee CS, Mendonça LS, et al. Model-to-data approach for deep learning in optical coherence tomography intraretinal fluid segmentation. JAMA Ophthalmol 2020; 138:1017-1024.
- Rieke N, Hancox J, Li W, et al. The future of digital health with federated learning. NPJ Digital Med 2020; 3:1-7.
- Chen C, Dou Q, Chen H, et al. Synergistic image and feature adaptation: Towards cross-modality domain adaptation for medical image segmentation. In Proceedings of the AAAI conference on artificial intelligence 2019; 33:865-872.
Indexed at, Google Scholar, Cross Ref
Indexed at, Google Scholar, Cross Ref
Indexed at, Google Scholar, Cross Ref
Indexed at, Google Scholar, Cross Ref
Indexed at, Google Scholar, Cross Ref
Indexed at, Google Scholar, Cross Ref
Indexed at, Google Scholar, Cross Ref
Indexed at, Google Scholar, Cross Ref
Indexed at, Google Scholar, Cross Ref
Indexed at, Google Scholar, Cross Ref
Indexed at, Google Scholar, Cross Ref
Indexed at, Google Scholar, Cross Ref
Indexed at, Google Scholar, Cross Ref
Indexed at, Google Scholar, Cross Ref
Indexed at, Google Scholar, Cross Ref
Indexed at, Google Scholar, Cross Ref
Indexed at, Google Scholar, Cross Ref
Indexed at, Google Scholar, Cross Ref
Indexed at, Google Scholar, Cross Ref
Indexed at, Google Scholar, Cross Ref
Indexed at, Google Scholar, Cross Ref
Indexed at, Google Scholar, Cross Ref
Indexed at, Google Scholar, Cross Ref
Indexed at, Google Scholar, Cross Ref
Indexed at, Google Scholar, Cross Ref
Indexed at, Google Scholar, Cross Ref
Indexed at, Google Scholar, Cross Ref
Indexed at, Google Scholar, Cross Ref
Indexed at, Google Scholar, Cross Ref
Indexed at, Google Scholar, Cross Ref
Indexed at, Google Scholar, Cross Ref
Indexed at, Google Scholar, Cross Ref
Indexed at, Google Scholar, Cross Ref
Indexed at, Google Scholar, Cross Ref
Indexed at, Google Scholar, Cross Ref
Indexed at, Google Scholar, Cross Ref
Indexed at, Google Scholar, Cross Ref
Indexed at, Google Scholar, Cross Ref
Indexed at, Google Scholar, Cross Ref
Author Info
Rukayya Muhammad1, Moussa Mahamat Boukar1, Steve Adeshina2 and Senol Dane3*
1Department of Computer Science, Faculty of Natural and Applied Sciences, Nile University of Nigeria, Abuja, Nigeria2Department of Computer Engineering, Faculty of Engineering, Nile University of Nigeria, Abuja, Nigeria
3Department of Physiology, College of Health Sciences, Nile University of Nigeria, Abuja, Nigeria
Citation: Rukayya Muhammad, Moussa Mahamat Boukar, Steve Adeshina, Senol Dane, Deep Learning-Based OCT for Epilepsy: A Review, J Res Med Dent Sci, 2022, 10 (8):39-46.
Received: 18-Jul-2022, Manuscript No. jrmds-22-69401; , Pre QC No. jrmds-22-69401 (PQ); Editor assigned: 20-Jul-2022, Pre QC No. jrmds-22-69401 (PQ); Reviewed: 04-Aug-2022, QC No. jrmds-22-69401; Revised: 09-Aug-2022, Manuscript No. jrmds-22-69401(R); Published: 16-Aug-2022