Research - (2022) Volume 10, Issue 3
Spatial and Space time Clustering of Diarrhoeal Cases among Under-Five Children in Karkala, Karnataka: A Geo-Spatial Analysis
Dmello MK1, Badiger S2*, Kumar S3, Kumar N4 and Dsouza N5
*Correspondence: Badiger S, Department of Community Medicine, AJ Institute of Medical Sciences, Mangaluru, India, Email:
Abstract
Globally, India tops by contributing the maximum number of diarrhoeal fatality and accounts for 13% of all deaths/year in children under-five years. Forecasting the path and spread of diarrhoeal disease is critical due to its multi-factorial cause, which needs robust spatial analysis and experiential investigations of communicable disease. Objectives: A retrospective longitudinal study was conducted in Karkala taluk of the Udupi district of Karnataka state, India, to investigate purely spatial, purely temporal, and space-time clusters of diarrhoea among under-five children using a geographic information system (GIS). Methods: The data on diarrhoea among under-five children was collected for three years, i.e. from April 1, 2015, to March 31, 2018, at the district health office. A total of forty-nine village data were obtained and about 3894 under-five childhood diarrhoea were reported during the study period. Results: The analysis of the spatial cluster using SatScan software for three years in the study area identified eight high-risk areas (p ≤ 0.0001), covering twenty-one villages. The most likely spatiotemporal cluster region was located at the northern Karkala, and the most-at-risk period was April 1, 2016, to September 30, 2017 (LLR=114.67 and p-value <0.00001). The analysis of purely temporal cluster showed that one most likely cluster happened in all villages (LLR=73.89, p<0.001) from April 1, 2017, to March 3, 2018. Conclusion: The prevention, control and health promotional activities can be planned using advanced spatial statistical methods. The spatial information will help the program planners to have horizontal and vertical equity.
Keywords
Diarrhoea, SatScan, GIS, Space-time clustering, Childhood, India
Introduction
Around 1.1 billion in the world people have no access to clean drinking water. Every year, four billion diarrhoea cases occur globally, and it is one of the severe problems in the developing world. Poor quality of water, sanitation, and hygiene are some of the leading cause of diarrheal incidence, and most deaths occur among underfive children due to diarrhoea [1]. Globally, India tops by contributing the maximum number of diarrhoeal fatality among under-five children and has become a leading cause of childhood death in India, accounting for 13% of all deaths/ year in children under five years [2,3]. Diarrhoea among under-five children not only varies across nations but also among different states of a given nation. These variations can be the result of climatic, environmental, behavioural, socioeconomic, and spatial factors.
The epidemiological study on infectious disease helps us identify the root of the disease, its progression, the role of the environment in the development of disease, and the outspread. Whenever the disease cause is multi-factorial and infectious, forecasting its spread becomes critical, which requires robust spatial analysis. Various aetiological hypotheses can be stated and tested by geographical mapping of the disease, which would help identify hotspot areas and policy advocacy. The spatial analysis also helps in identifying the spatial-temporal cluster of the particular geographic area [4,5].
Udupi district is located in the Karnataka state of Indian nation has also contributed to diarrheal disease among Under-five children. According to District Health office sources, Udupi district (unpublished source) out of 84,246 under-five children, 8486 children had a diarrheal episode. According to DLHS 4, the burden of diarrheal disease in the Udupi district has doubled (4.2%) more than twice compared to that of DLHS 3 (1.9%) [6]. This study's main objective is to investigate purely spatial, purely temporal, and space-time clusters of diarrhoea among underfive children using geographic information system (GIS) in Karkala taluk of Udupi district.
Materials and Methods
A retrospective longitudinal study was conducted in Karkala block/taluk of the Udupi district in the year 2019-2020. The study area located in the south-west part of the country between 13011’60’’ North latitude and 74058’48’’ East longitude. A geographic location represented each village by the geo-coordinates taken from a village's representative site. Geo-coordinates were précised by means of a standard Cartesian coordinate system. The Karkala block has three seasons: summer, monsoon and winter. The warm season starts from March to May, monsoon from June to August and winter from September to February. In the region of study, the average highest and lowest temperatures are 260C and 110C, respectively.
The cases were operationally defined as the number of under-five children identified with diarrhoea in all study villages healthcare facility. The data on diarrhoea among under-five children was collected for three years, i.e. from April 1, 2015, to March 31, 2018, at the district health office by reviewing monthly reports made to the regular surveillance system. To validate diarrhoea's information among under-five children at the village level, the researcher implemented a top to bottom approach, i.e. District level – taluk level- primary health centre – subcenter. The demographic data of 49 villages were extracted from the subcenter and validating it at each village panchayath. The trained health professionals collected the data.
Data analysis
The annual under-five children diarrhoea incidences per 1000 under-five population at risk in each village from April 2015 to March 2018 were calculated using excel. The excess hazard ratio (standard morbidity ratio >1) for every village was estimated by dividing the observed cases by expected cases. The expected number of cases in each village was estimated by E(c)=p*C/P, where c is the number of expected cases, and p is the population of the study village, C is the total number of observed cases, and P is the total population in study taluk [7].
Cluster analysis
The Spatiotemporal and cluster analysis was conducted in 49 villages using the Kulldorff scan statistic (SaTScan v9.6.1). The SatScan software was used to detect the randomness of diarrhoea distribution over space and time among underfive children. By considering the number of observed and expected cases inside the window, the software identifies and assesses the statistical significance of spatial or space-time clusters. The scanning window in SaTScan software considers time (interval), space (circle/ellipse) and spacetime (a cylinder with an elliptical or circular base). A discrete Poisson-based model was used to analyse the monthly reported diarrhoeal cases following Poisson distribution [8].
Purely spatial clusters
For identifying spatial clusters of under-five children with diarrhoea, a purely spatial analysis was performed using SatScan software without taking time into account. For analysing the spatial statistics, the SaTScan software uses a circular window that scans the entire study area. The circle radius will take different values ranging from zero to the mentioned largest size. This fixed highest size determines the total population of under-five children at risk within the scanning window. It is recommended that the largest size should not be beyond 50%, i.e. the identified cluster will have no more than 50% of the under-five children at risk [9]. The spatial scan statistic's null hypothesis states that diarrhoea among under-five children is randomly distributed throughout the villages and that the expected event count is proportional to the population at risk. If the null hypothesis is statistically rejected for any circular window, then the scan window's geographic area can be considered a spatial cluster. StatScan software uses a Monte Carlo simulation to test the assumption for each location and size of the scanning window. The null hypothesis states that there is no significant difference in observed cases inside and outside the scanning window for each circle. The likelihood ratio is calculated within each process, assuming Poisson distribution. The likelihood function for a specific window is calculated using:
[c/E(C)] [C-c/C-E(c)] I[c>E(c)]
iWhere c is the number of under-five children with diarrhoea observed inside the window (village), and E(c) is the indirectly age-adjusted expected number of under-five children with diarrhoea inside the window (in that village), C is the total number of under-five children in the study area, C-c and C-E(c) are proportional to the age-standardized incidence ratios within and outside the window respectively, and I is an indicator function, I = 1, when the window has more diarrhoeal cases than expected.
The most likely cluster is identified by the likelihood ratio having a higher value and with p-value significance. The Monte Carlo simulations (999) technique is used to estimate the p-value [9]. The significant cluster was identified with a p-value <0.05.
Spatiotemporal and temporal clusters
To detect temporal clusters with high rates of diarrhoea, a purely temporal cluster analysis was performed. The upper limit size of the cluster was set to 50% of the population at risk within the study period. The most likely cluster was identified by the likelihood ratio having the highest value. The p ≤ 0.05 was considered to be significant.
Space-time scan statistic was used to detect clusters both in space and time. The spacetime scan statistic uses a cylindrical window with a circular (or elliptic) geographic base and height related to time to determine spatiotemporal clusters. The purely spatial scan statistic is reflected by the geographic base of the cylindrical window, while the height of the cylindrical window reflects the time period. For each possible geographical location and size, the cylindrical window is moved in both space and time; it also visits each possible time interval. For each space-time window, the likelihood ratio is calculated to determine the rate of observed cases and expected cases. The Monte Carlo simulations (999) technique is used to estimate the p-value [10].
The study was approved by the Central Ethics Committee [Ref: NU/CEC/2019/0212, Date of approval: 30.01.2019]. In addition, formal approval was also taken from the District Health Officer of Udupi.
Results
A total of fifty village data were obtained, and about 3894 under-five childhood diarrhoea were reported from April 1, 2015, to March 31, 2018. The total under-five population of villages in Karkala taluk for 2015-2016, 2016-2017 and 2017-2018 were 12,038, 12,116 and 11,848, respectively. In Karkala taluk, the combined incidence rate of diarrhoea was 123.6 cases per 1000 under-five population at risk. The overall incidence rate of diarrhoea among girl children was 385.7 per 1000 girl child, while the incidence rate for boys was 362.5 per 1000 boys. The maximum incidence of diarrheal cases (672 per 1000 population) was seen in the second year (13 to 24 months), while least (148.6 per 1000) was seen in children below six months of age. The highest incidence of diarrhoea was seen in April (650 per 1000 population) followed by July (350 per 1000 population), and least was seen in November (15.8 per 1000 population at risk) (Table 1).
Variables | Incidence rate per 1000 under-five population | |||||||||||
---|---|---|---|---|---|---|---|---|---|---|---|---|
2015-16 | 2016-17 | 2017-18 | Overall | |||||||||
Mean | SD | Max. | Mean | SD | Max. | Mean | SD | Max. | Mean | SD | Max. | |
Overall | 100.6 | 75.5 | 294.1 | 114.2 | 82.1 | 299.2 | 159.3 | 116.9 | 652.2 | 123.6 | 83.1 | 360.5 |
Sex | ||||||||||||
Boy | 96.4 | 82.1 | 407.4 | 110.1 | 83.4 | 333.3 | 167.9 | 123.4 | 666.7 | 123.2 | 82.9 | 362.5 |
Girl | 106.1 | 85.3 | 307.7 | 120 | 90 | 327.9 | 152.5 | 117.4 | 636.4 | 125 | 86.8 | 385.7 |
Age (in months) | ||||||||||||
<6 | 28.4 | 62.5 | 272.7 | 35.6 | 84.2 | 388.9 | 20.7 | 57.2 | 333.3 | 25.4 | 40.7 | 148.6 |
7-12 | 109.5 | 131.2 | 428.6 | 169.6 | 170.5 | 533.3 | 146.4 | 197.9 | 750 | 142.5 | 124.2 | 473.7 |
13 - 24 | 281.5 | 217.8 | 857.1 | 255.7 | 187.7 | 705.9 | 266.7 | 202.7 | 1000 | 272.1 | 128.5 | 672.1 |
25 - 36 | 254.5 | 197.3 | 1000 | 232.4 | 171.9 | 666.7 | 222.2 | 151.7 | 692.3 | 232.6 | 109.5 | 487.8 |
37 - 48 | 231.1 | 212.1 | 857.1 | 211.5 | 197.7 | 666.7 | 194 | 194.9 | 1000 | 201.8 | 135.3 | 631.6 |
49 - 60 | 95 | 141.5 | 666.7 | 95.1 | 117.3 | 407.4 | 150 | 221.5 | 1000 | 125.5 | 121.4 | 526.3 |
Month | ||||||||||||
Jan | 78.7 | 95.8 | 333.3 | 38.4 | 49.9 | 222.2 | 61.7 | 69.3 | 250 | 57.1 | 50.3 | 194.4 |
Feb | 77.6 | 95.6 | 333.3 | 59.6 | 89.6 | 333.3 | 69.9 | 81.9 | 333.3 | 64 | 47.7 | 216.7 |
Mar | 156.5 | 242.8 | 1000 | 122.4 | 159.7 | 687.5 | 98.6 | 106.9 | 500 | 115.2 | 80.1 | 371.4 |
April | 95.1 | 122.6 | 500 | 113.1 | 161.7 | 1000 | 140.9 | 192 | 1000 | 124.6 | 122.7 | 650 |
May | 73.2 | 101.6 | 500 | 74.8 | 78.9 | 333.3 | 78.7 | 91.3 | 391.3 | 78.8 | 52.8 | 250 |
June | 132.9 | 145.5 | 666.7 | 87.3 | 147.3 | 1000 | 62.7 | 67.5 | 304.4 | 88 | 55.2 | 258.1 |
July | 91.9 | 80.2 | 350 | 116.9 | 155.3 | 666.7 | 125.6 | 129.9 | 684.2 | 116.5 | 80 | 350 |
August | 65.7 | 75.9 | 285.7 | 115.2 | 128.4 | 750 | 87.2 | 75.1 | 333.3 | 88.2 | 42.45 | 210.5 |
September | 52.5 | 56.2 | 181.8 | 69 | 63.1 | 166.7 | 65.7 | 68.6 | 312.5 | 67.9 | 41.8 | 161.3 |
October | 61.9 | 83.5 | 428.6 | 62.8 | 66.6 | 285.7 | 69.7 | 69.3 | 333.3 | 66.3 | 51.8 | 210.5 |
November | 44.9 | 60.2 | 250 | 67.8 | 81.5 | 363.6 | 71.6 | 82.8 | 366.7 | 62.4 | 43.8 | 153.8 |
December | 69.1 | 74.2 | 333.3 | 72.7 | 98.3 | 476.2 | 67.8 | 65.9 | 233.3 | 70.9 | 59.9 | 266.7 |
Table 1: Distribution of diarrhoeal incidence among under-five children w.r.to sex, age and month in karkala taluk.
The Standard Morbidity Ratio (SMR) was determined by the number of observed cases over the number of expected cases. Almost 50% of villages had SMR greater than one, seven villages had greater than two, while fifteen villages had SMR greater than one (Figure 1).
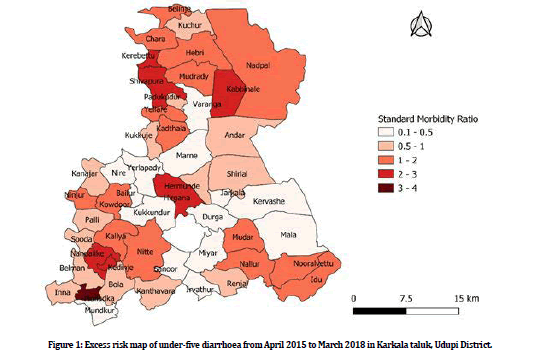
Figure 1: Excess risk map of under-five diarrhoea from April 2015 to March 2018 in Karkala taluk, Udupi District.
The diarrhoea among under-five children varied considerably across the villages. Overall, twenty-four villages had incidence rates of 100 or more cases per 1000 under-five children at risk. The Mulladka village witnessed the highest incidence rate (652.2 cases per 1000 children) in 2017-2018, while the lowest incidence was seen in Sanoor village (6.8 per 1000 children) in 2015-2016. The Mulladka village saw the overall highest incident cases (360.5 per 1000 underfive children at risk) and the least cases (19.5 per 1000 under-five children at risk) in Sanoor village.
For the period of three years, the spatial clustering analysis showed a total of eight statistically significant high-risk areas. The identified high-risk areas covered a total of twenty-one villages. The Global Moran's I values ranged from 0.016 to 0.351 for every year, with a significant p-value suggesting positive spatial autocorrelation. The most likely cluster with a relative risk of 2.03 was observed in northern Karkala. The cluster window of the most likely cluster was centred at 74.971980 N, 13.505510 E (LLR = 137.76, P<0.0001) with a relative risk of 1.87, an observed number of 1083 cases, and an expected 665.9 cases. The circular area covered ten villages with a radius of 4.64 Km, including Belinje, Chara, Shivapura, Kerebettu, Kuchur, Padukudoor, Yellare, Hebri, Mudradi, Kadthala. The relative risk of secondary clusters within a non-random distribution pattern was also significant (p<0.001) (Table 2).
Cluster type | Coordinates/Radius | Cluster Village | Observed Cases | Expected Cases | RR | LLR | P-Value |
---|---|---|---|---|---|---|---|
Primary Cluster | 13.489810 N, 75.001120 E/11.45 km | Kuchur, Belinje, Hebri, Chara, Kerebettu Mudradi, Shivapura, Nadpal, Kabbinale | 1185 | 688.14 | 2.04 | 187.867 | <0.00001 |
Secondary cluster | 13.170210 N, 74.893430 E/1.44 km | Kedenje, Nandalike | 201 | 76.2 | 2.73 | 72.21 | <0.00001 |
Secondary cluster | 13.265440 N, 74.983880 E/0 km | Hirgana | 226 | 103.98 | 2.25 | 55.41 | <0.00001 |
Secondary cluster | 13.149560 N 75.159090 E,/2.93 km | Idu, Nooralettu | 259 | 139.01 | 1.92 | 43.11 | <0.00001 |
Secondary cluster | 13.130840 N, 74.867320 E/0 km | Mulladka | 53 | 16.14 | 3.32 | 26.32 | <0.00001 |
Secondary cluster | 13.170060 N 75.079280 E/0 km | Nallur | 204 | 133.85 | 1.55 | 16.47 | 0.00001 |
Secondary cluster | 13.186290 N 74.942300 E/0 km | Nitte | 274 | 209.18 | 1.33 | 9.716 | 0.0018 |
Table 2: Spatial clustering of diarrhoea among under-five children in Karkala taluk, India, 2015-2018.
One most likely cluster and three secondary clusters were identified by keeping the maximum spatial cluster size as 50% of the total population. The most likely Spatio-temporal cluster area was located at the northern Karkala, and the highrisk period was April 1, 2016, to September 30, 2017 (LLR =114.67 and p-value <0.00001). The centre of the most likely cluster was in Kuchur village, 13.489810 N, 75.001120 E, covering nine villages: Kuchur, Belinje, Hebri, Chara, Kerebettu, Mudradi, Shivapura, Nadpal, Kabbinale with a radius of 11.45 km. During this period, a total of 641 diarrhoeal cases among under-five children were reported with a relative risk of 2.03. The first spatiotemporal secondary cluster area was located at the south-west of Karkala. The increased risk period for diarrhoea among under-five was March 1, 2017, to September 30, 2017 (LLR= 74.372, p<0.00001). The first secondary cluster centre was in Sooda village, 13.203370 N, 74.858880 E, covering 13 villages, namely Sooda, Palli, Nandalike, Kallya, Belman, Kedenje, Ninjur, Kowdoor, Mulladka, Bola, Inna, Nitte and Kanajar. Altogether 325 diarrhoeal cases were reported during the high-risk period with a relative risk of 2.19. In Karkala taluk, March to May and June to September are hot weather periods and south-west monsoons (Figure 2).
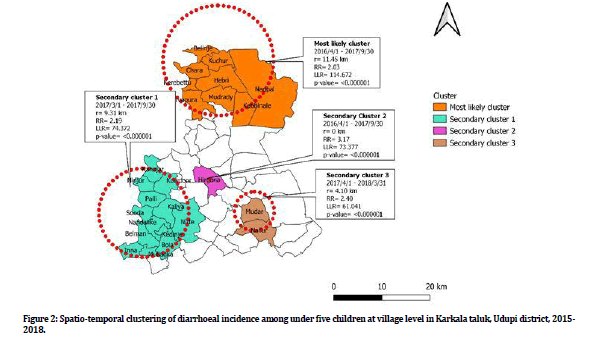
Figure 2: Spatio-temporal clustering of diarrhoeal incidence among under five children at village level in Karkala taluk, Udupi district, 2015-2018.
Purely temporal clusters of childhood diarrhea
All villages witnessed one most likely cluster in the month of April 1, 2017, to March 3, 2018 (LLR=73.89, p<0.001). The overall RR within the cluster was 1.49 (p<0.0001), with an observed number of 1661 and 1296.4 expected cases. There were no secondary clusters identified.
Discussion
The study analysed the spatial and space-time distribution of under-five diarrhoea in Karkala taluk from April 2015 to March 2018 using Kulldorff’s scan statistical analysis. The study established significant space-time clustering of diarrhoea incidents among under-five in Karkala taluk.
The study exhibited seasonal variation of diarrhoea among under-five children. The incidence rate was highest in Mulladka village for the year 2017/18. The high-risk space-time spots of diarrhoea were observed in southwest Karkala. The findings were consistent with the study conducted in Chennai, showing that climatic factors like high temperature and heavy rainfall were significantly associated with childhood diarrhoea [11-14]. The high temperature helps in bacterial growth and favours its survival in the external environment [13,15,16]. The flooding and surface run water will enhance drinking water source contamination during the rainy season [17]. The dry seasons lead to increase consumption of water because of high temperatures. Unhygienic drinking water practices and water scarcity leads to the transmission of diarrhoea [17,18]. Most of the household wells and bore wells will be dried out during the summer season, making households use unimproved water sources [17].
One of this study's critical finding is the nonrandom spatial distribution of diarrhoea in Karkala village. In the northern zone of Karkala taluk, a purely spatial cluster was identified. The spatiotemporal models indicated the most likely cluster was located in the northeast area of Karkala taluk. The first secondary clusters were found in thirteen villages of the south-west zone and others in the southeast zone of Karkala taluk. The spatiotemporal clusters witnesses' relatedness in geographical parameters like climate conditions, altitude and socio-demography. In epidemiology, cluster analysis's importance is to detect cases' aggregation and eventually find the evidence of risk factors. Disease prevention, protection, and health promotion activities can be planned using cluster analysis [19].
The excess risk places of diarrhoea cases in the study area are seen within a defined place and time. The most likely spatiotemporal cluster was found in all northern Karkala and the south-west region between April 1, 2016, and September 30, 2017. This might be due to the precipitation changes over India's years, leading to climate change [20]. Significant high rates of purely temporal under-five diarrhoea cases were detected in all villages from April 1, 2017, to March 3, 2018. This could be due to the decreasing trend in south-west monsoon rainfall and a slight increase in north-east monsoon in the year 2017/18 [21,22].
The strength of the study is the complete line listing of cases day-wise was obtained for all the villages from the district health office and validating at the sub-centre level. The other strength was on-time reporting of diarrhoea cases by the accredited social health activist (ASHA) by conducting house to house visits. SaTScan software's use helped us detect local clusters with good accuracy and evaluate statistical significance without any trouble. A limitation is under-reporting by the guardians of under-five children during active surveillance conducted by ASHA workers.
Conclusion
There was significant spatial and spatiotemporal clustering of diarrhoeal cases among underfive children in Karkala taluk. The Karkala taluk also saw the seasonal distribution of underfive diarrhoea cases during 2015-2018. The prevention, control and health promotional activities can be planned using advanced spatial statistical methods. The spatial information will help the program planners have equity in resource distribution, such as horizontal equity and vertical equity. The health authorities should ensure the execution of specific intervention like potable water supply, healthy environment and hygienic practices, drainage facility, and good food safety and water treatment practices for appropriate locations.
Acknowledgement
We thank the Medical officers and community health workers of the PHCs for granting us the permission and helping in data collection process. Necessary permissions were obtained from the District Health Office and Central Ethics Committee, Nitte (Deemed to be University) [Ref: NU/CEC/2019/0212, Date of approval: 30- 01-2019] before the conduct of the study.
Ethical Issues
The authors hereby certify that all ethical issues were considered while collecting the data, and results from the study have not been published elsewhere. The study was approved by the Central Ethics Committee [Ref: NU/ CEC/2019/021]. The necessary permission from the District Health Authority and informed consent from participant and the guardians who participated in the study was undertaken.
Authors Contributions
All authors have contributed to data collection and analysis, and paper writing.
Disclosure Statement
The authors reported no potential conflict of interest.
References
- Bessong PO, Odiyo JO, Musekene JN, et al. Spatial distribution of diarrhoea and microbial quality of domestic water during an outbreak of diarrhoea in the Tshikuwi community in Venda, South Africa. J Health Popul Nutr 2009; 27:652.
- Lakshminarayanan S, Jayalakshmy R. Diarrheal diseases among children in India: Current scenario and future perspectives. J Nat Sci Biol Med 2015; 6:24–8.
- Kumar VS, Devika S, et al. Spatial mapping of acute diarrheal disease using GIS and estimation of relative risk using empirical bayes approach. Clin Epidemiol Glob Health 2016;
- Saravana Kumar V, Devika S, George S, et al. Spatial mapping of acute diarrheal disease using GIS and estimation of relative risk using empirical Bayes approach. Clin Epidemiol Glob Health 2017; 5:87–96.
- Waller LA, Carlin BP, Xia H, et al. Hierarchical spatio-temporal mapping of disease rates. J Am Stat Assoc 1997; 92:607–617.
- http://www.rchiips.org/DLHS4.htm
- Reiner RC, Wiens KE, Deshpande A, et al. Mapping geographical inequalities in childhood diarrhoeal morbidity and mortality in low-income and middle-income countries, 2000–17: Analysis for the global burden of disease study 2017. Lancet 2020; 395:1779–801.
- Kulldorff M. A spatial scan statistic. Commun Stat Theory Methods 1997; 26:1481–96.
- Kulldorff M, Nagarwalla N. Spatial disease clusters: Detection and inference. Stat Med 1995; 14:799–810.
- Kulldorff M. An isotonic spatial scan statistic for geographical disease surveillance. J Natl Inst Public Health 1999; 48:94–101.
- Mertens Andrew, Balakrishnan Kalpana, Ramaswamy Padmavathi, et al. Associations between High temperature, heavy rainfall, and diarrhea among young children in rural Tamil Nadu, India: A prospective cohort study. Environ Health Perspect 2019; 127:047004.
- Bado AR, Susuman AS, Nebie EI. Trends and risk factors for childhood diarrhea in sub-Saharan countries (1990–2013): Assessing the neighborhood inequalities. Glob Health Action 2016; 9:30166.
- Zhou X, Zhou Y, Chen R, et al. High temperature as a risk factor for infectious diarrhea in Shanghai, China. J Epidemiol 2013.
- Levy K, Woster AP, Goldstein RS, et al. Untangling the impacts of climate change on waterborne diseases: A systematic review of relationships between diarrheal diseases and temperature, rainfall, flooding, and drought. Am Chem Society 2016; 50:4905-22.
- Bentham G, Langford IH. Environmental temperatures and the incidence of food poisoning in England and Wales. Int J Biometeorol 2001; 45:22–26.
- Islam MS, Sharker MAY, Rheman S, et al. Effects of local climate variability on transmission dynamics of cholera in Matlab, Bangladesh. Trans R Soc Trop Med Hyg 2009; 103:1165–70.
- Hoque BA, Hallman K, Levy J, et al. Rural drinking water at supply and household levels: Quality and management. Int J Hyg Environ Health 2006; 209:451–60.
- Chou WC, Wu JL, Wang YC, et al. Modeling the impact of climate variability on diarrhea-associated diseases in Taiwan (1996–2007). Sci Total Environ 2010; 409:43–51.
- Odoi A, Martin SW, Michel P, et al. Investigation of clusters of giardiasis using GIS and a spatial scan statistic. Int J Health Geogr 2004; 3:11.
- Kulkarni A, Sabin TP, Chowdary JS, t al. Precipitation Changes in India. In: Krishnan R, Sanjay J, Gnanaseelan C, et al. Assessment of climate change over the Indian region: A report of the ministry of earth sciences (MoES), Government of India. Singapore: Springer 2020.
- https://des.kar.nic.in/sites/Annual%20Rainfall-2018.pdf
- Pai MS. A descriptive study to assess the knowledge and practice regarding water, sanitation and hygiene among women in selected villages of Udupi district. Nitte Univ J Health Sci 2016; 6:21.
Indexed at, Google Scholar, Cross Ref
Indexed at, Google Scholar, Cross Ref
Indexed at, Google Scholar, Cross Ref
Indexed at, Google Scholar, Cross Ref
Indexed at, Google Scholar, Cross Ref
Indexed at, Google Scholar, Cross Ref
Indexed at, Google Scholar, Cross Ref
Indexed at, Google Scholar, Cross Ref
Indexed at, Google Scholar, Cross Ref
Indexed at, Google Scholar, Cross Ref
Indexed at, Google Scholar, Cross Ref
Indexed at, Google Scholar, Cross Ref
Indexed at, Google Scholar, Cross Ref
Indexed at, Google Scholar, Cross Ref
Indexed at, Google Scholar, Cross Ref
Indexed at, Google Scholar, Cross Ref
Author Info
Dmello MK1, Badiger S2*, Kumar S3, Kumar N4 and Dsouza N5
1Department of Public Health, K S Hegde Medical Academy, Nitte (Deemed to be University), Mangaluru, India2Department of Community Medicine, AJ Institute of Medical Sciences, Mangaluru, India
3Senior State Consultant, Karnataka, UNICEF, India
4Department of Community Medicine, K S Hegde Medical Academy, Nitte (Deemed to be University), Mangaluru, India
5Department of Statistics, K S Hegde Medical Academy, Nitte (Deemed to be university), Mangaluru, India
Citation: Dmello MK, Badiger S, Kumar S, Kumar N, Dsouza N, Spatial and Space time Clustering of Diarrhoeal Cases among Under-Five Children in Karkala, Karnataka: A Geo-Spatial Analysis, J Res Med Dent Sci, 2022, 10 (3):20-27.
Received: 01-Feb-2022, Manuscript No. JRMDS-22-53021; , Pre QC No. JRMDS-22-53021 (PQ); Editor assigned: 03-Feb-2022, Pre QC No. JRMDS-22-53021 (PQ); Reviewed: 17-Feb-2022, QC No. JRMDS-22-53021; Revised: 22-Feb-2022, Manuscript No. JRMDS-22-53021 (R); Published: 01-Mar-2022